[see also this short slide presentation]
We aim to apply ReComp concepts and methods to specific concrete problems in genomics and metagenomics.
NGS (WES/WGS) analysis pipelines have multiple “moving parts” that
evolve and change over time: software tools as well as reference
datasets.
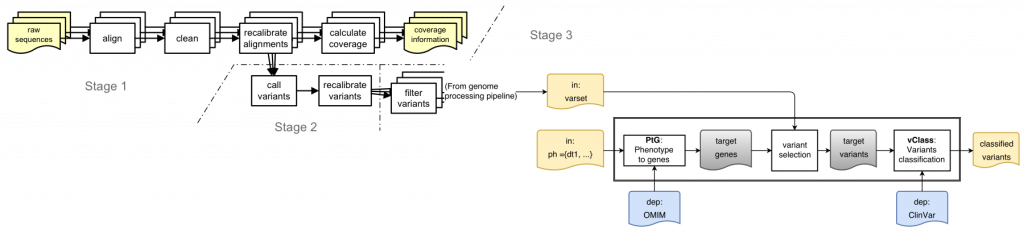
Regarding genomics, we want to address the following practical problem.
Suppose we maintain a population of patients along with their analysis
results (variants, variant interpretation, ….) obtained in the past,
under known pipeline configuration.
Any change in any of the libraries, software packages, or reference
datasets used in any of these pipelines is likely to have some impact on
element of the population. This can be measured for instance in terms
of likelihood of changes in a patient’s diagnosis.
In the event of a change, we could blindly re-analyse the entire
population. However this is likely to be inefficient when the change has
low impact on the population. Thus, we would like to develop techniques
for predicting and estimating the extent of the impact, so that re-analysis can be prioritised given a fixed budget.
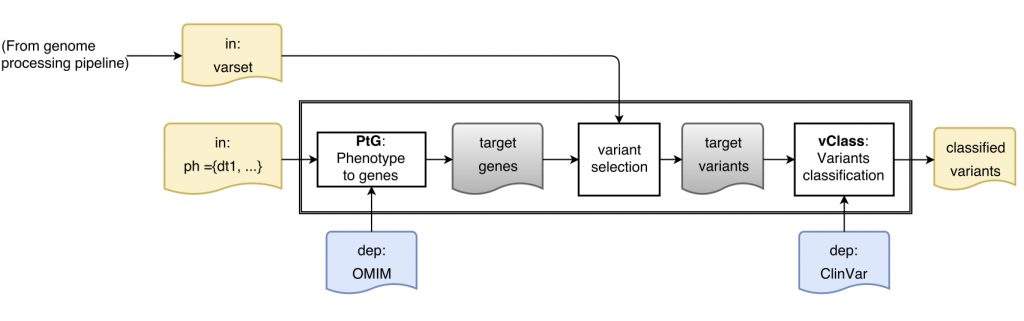
Is this a real problem? Here is a sketch of a simple variant interpretation workflow that makes use of OMIM GeneMap and CLinVar to determine pathogenicity of variants that are relevant for a patient with a given phenotype:
[Learn more about ReComp in the context of the Simple Variant Interpretation pipeline]
And here below is a chart to show the impact of updated to ClinVar, during a short window of less than a year, on a very small cohort of patients, grouped by phenotype. The circles indicate that variants with uncertain pathogenicity have been either added or removed, while the X indicates that one or more off the variants have been identified as deleterious. These are high impact changes, which may affect a patient’s diagnosis.
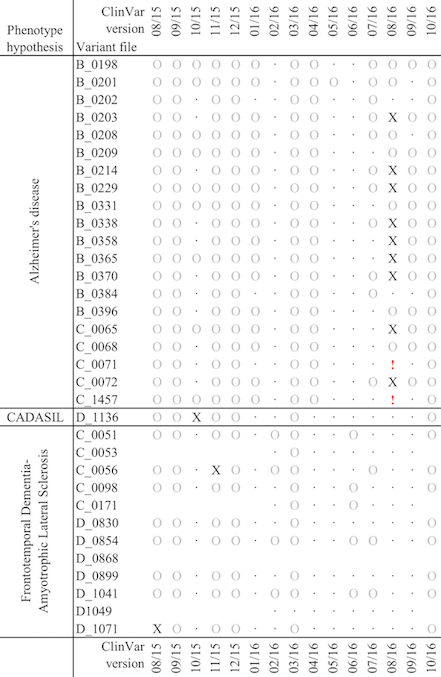