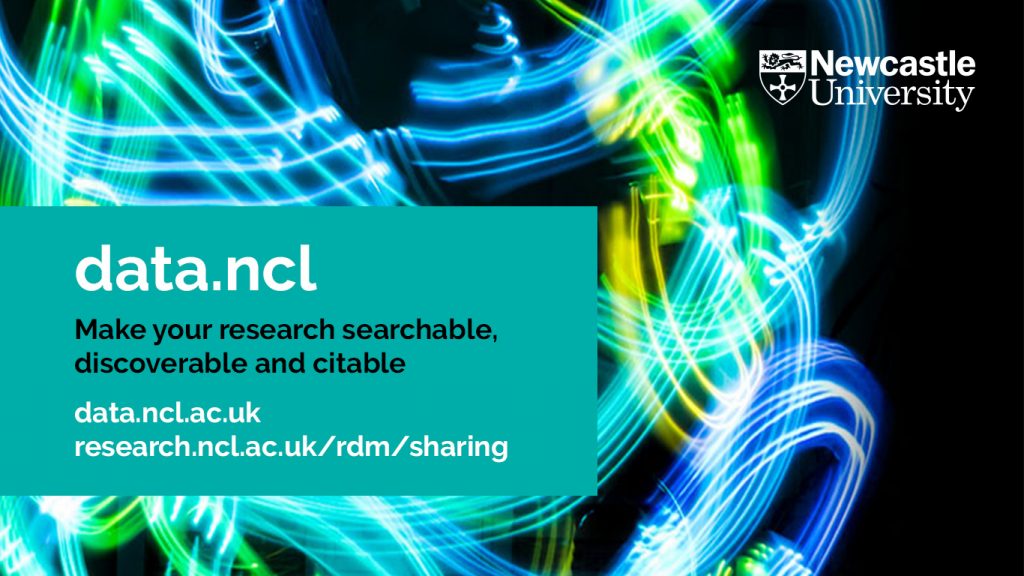
During the summer months data.ncl, Newcastle’s research data repository, reached over 500,000 views from datasets and code archived from researchers across the University. Reaching this milestone affords us the opportunity to take stock of how far we have travelled in openly sharing data as data.ncl was only launched in spring 2019.
It equally provides an indication of the reach data can have when it is archived and becomes findable, searchable and citable. Records in data.ncl have been viewed from as far away as Chile and New Zealand while the three countries who view and access data most frequently are USA, Netherlands and the UK – showing the global and national interest in research created at Newcastle University. In addition to views, data.ncl has enabled 215,000 downloads and preserves over 1200 records for future reuse.
“The long-term archiving and sharing of datasets through data.ncl is a significant part of our support for Open Research. Seeing datasets being viewed, accessed and reused shows there is real value in giving data a second life through data.ncl” said Professor Candy Rowe, Dean for Research Culture and Strategy. Professor Brian Walker, Pro-Vice-Chancellor for Research Strategy and Resources added: “Reaching this milestone shows Newcastle University is committed, along with UK government and other research funders, to the conduct of Open Research that is available to and used by as many people as possible for as long as possible”.
All researchers and PGRs can freely archive and publicly share data from their research through data.ncl. Archived data obtains its own DOI (Digital Object Identifier) for inclusion in research outputs, including publications. To help increase engagement and impact archived data is indexed by Google Scholar and Google Dataset search. Data collections can also be created to group together data records produced from a project or research theme with its own DOI to increase discovery. This can include records of data held in discipline specific repositories to create a full showcase of the data produced by a research project.
The Research Data Service has reviewed and approved hundreds of datasets and these are a few highlights:
- The Coral Spawning Database brings together a huge international effort that includes over 90 authors from 60 institutions in 20 countries to openly share forty years of coral data in one place for the first time. The intention is for this database to grow over time so the data isn’t set in stone and can be added to as the research progresses. Dr James Guest said: “Coral reefs have been declining in health for decades and are severely threatened by climate change. It is, therefore, more important than ever to share large datasets on these ecosystems so that they can be used to guide management of reefs in the Anthropocene”. James added: “When we were looking for a suitable data repository for the Coral Spawning Database, data.ncl was the obvious choice because it was so user friendly and has excellent support from the Research Data Service at Newcastle University”.
- Through National Lottery Heritage Fund, Dr Nicky Garland mapped and shared a number of features of Hadrian’s Wall including forts, towers, and road systems. The aim was to make the data open and accessible to allow researchers and the wider community to engage with Hadrian’s Wall and its conservation and research. The data records are proving to be very popular and are clearly supporting the aims of the WallCAP project. “In terms of our project decision to use data.ncl – it was a no-brainer! WallCAP will generate a considerable amount of data and we want that data to be readily accessible. Having a secure digital archive that provides DOIs that can be easily incorporated into academic publications is not only convenient, but essential in this era of data-proliferation” said Dr Rob Collis, Project Manager.
- The Dental Micromotor Handpiece Dataset was one of the first open data examples of Newcastle University responding to the Covid-19 Pandemic. James Allison, Clinical Fellow, explained: “Our project looked at how we can use novel dental drill designs to reduce the amount of aerosol produced during dental procedures. This is important because concerns over transmitting viruses in these aerosols caused dental services to shut down during the Covid-19 pandemic. Our work showed that these drills produce less aerosol and therefore reduce this risk, allowing them to be safely used in dental practices. This also helped dental students get back to treating their patients at the School of Dental Sciences and in other institutions in the UK. We felt it was important to share our data on data.ncl so that it was available to other researchers looking at the same problem, and also to those developing guidance and policy documents to inform their decisions.”
- Ali Alammer was a PhD researcher who shared his underpinning code for a biologically inspired machine vision model (En-HMAX), which rapidly processes 2D images with minimal computational requirements. Ali explained: “With a hierarchy of only six processing layers, the model was capable of extracting formative and unique representation to objects and scenes. It had also achieved comparable performances to existing state-of-the-art architectures including deep learning. I archived the code for research reproducibility purposes as it has a wide range of applications that includes surveillance and robotic vision.”
The Research Data Service runs data.ncl and supports researchers in planning, managing and sharing research data. For further information please visit the research data management website or contact rdm@ncl.ac.uk.